Latest News
Innovative Method Enhances Bridge Damage Detection Using Acceleration Trendlines
By analyzing acceleration responses, researchers have devised an innovative way to detect structural damage in bridges, which is a big step forward in structural health monitoring. This mechanism extrapolates trendlines for acceleration data using quadratic regression and is, therefore, much better than techniques based on filters such as moving average, Savitzky–Golay, and so forth.
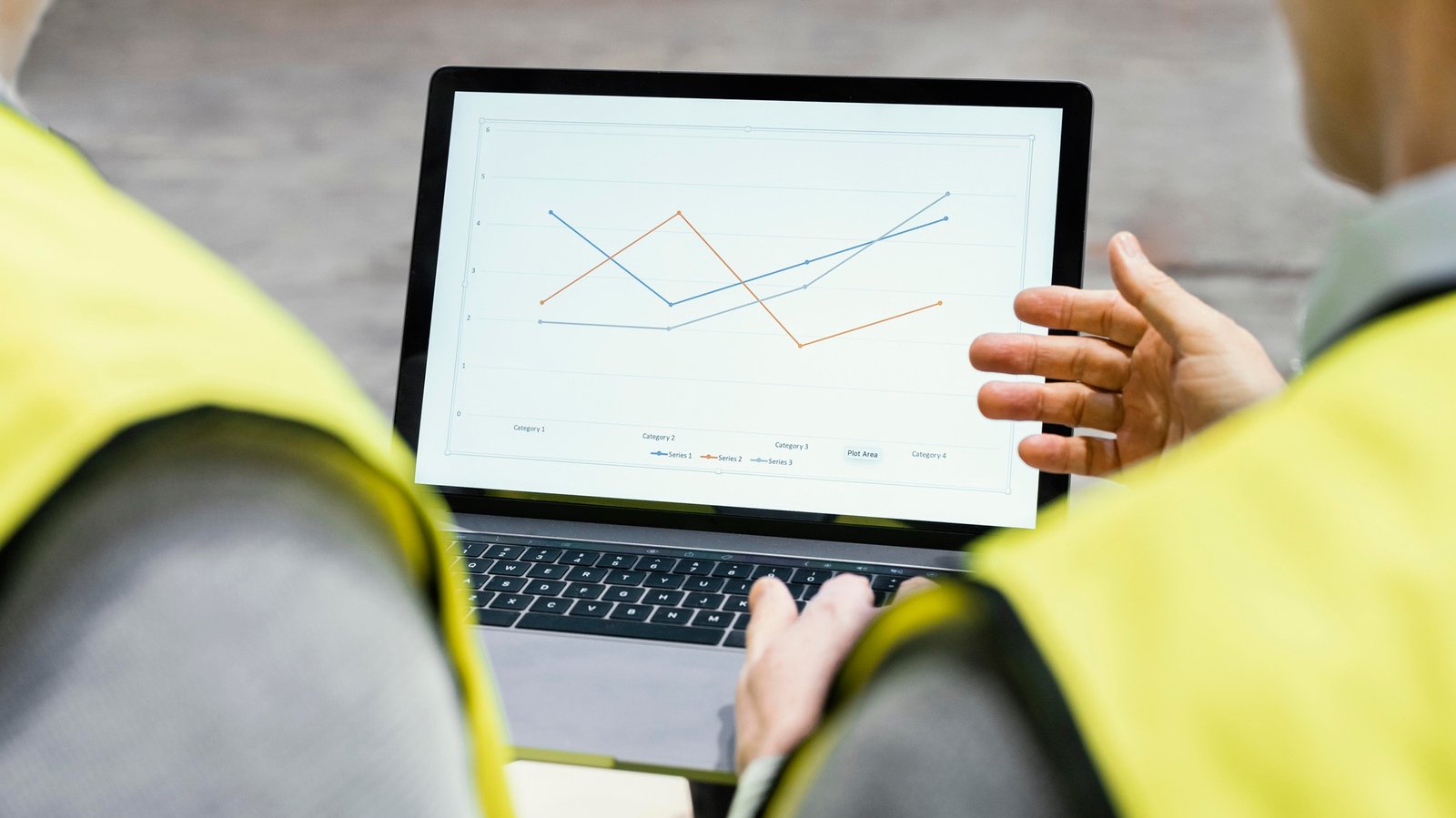
Enhanced Structural Damage Detection with PSO and Dynamic Time Wrapping
A groundbreaking methodology for damage detection and localization in structural systems has emerged, blending vibration-based techniques with Finite Element Models (FEM) and metaheuristic optimization algorithms. This innovative approach addresses a critical challenge: the modeling discrepancies between physical structures and their corresponding FE models, crucial for precise damage assessment in complex structures.
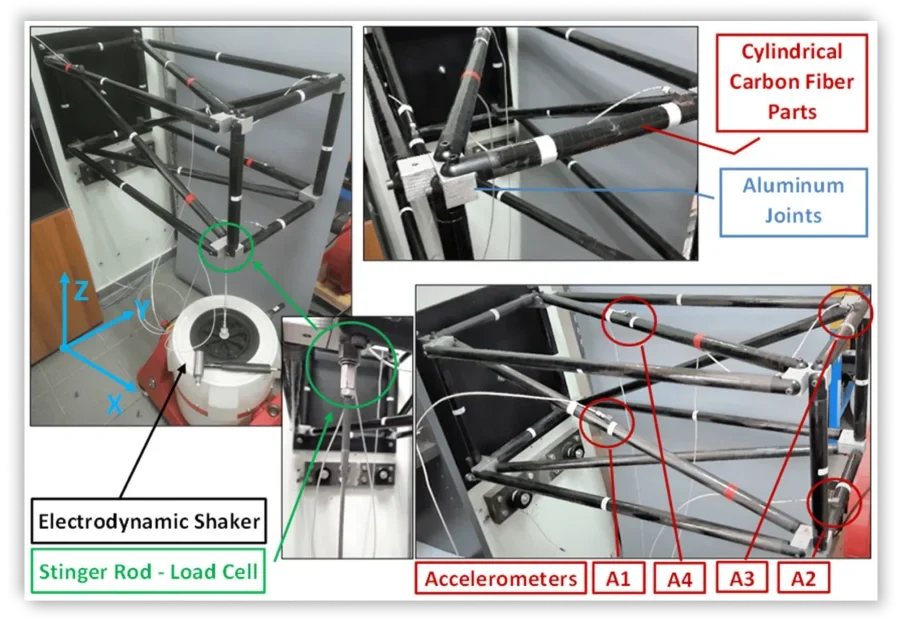
Advanced Method Revealed for Non-Contact Measurement of Mechanical Vibrations and Displacements Using Deferred Moiré Patterns
A groundbreaking technique for measuring mechanical vibrations and displacements without physical contact has been introduced in a recent paper published in “Advances in Mechanical Engineering.” Termed “deferred moiré patterns,” this method captures vibrations using a video record rather than simultaneous observation during field measurements. The innovation lies in filming a vibrating grid attached to an object, enabling subsequent analysis.
Central to this approach are two principles: moiré magnification and moiré phase. The magnification ensures the precise measurement of minute quantities, even below pixel level.
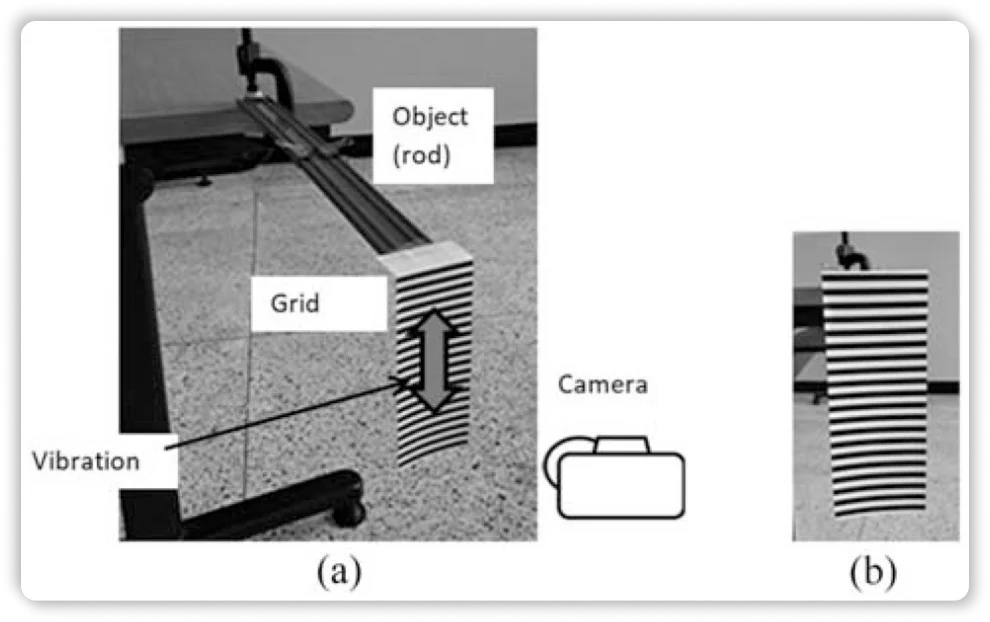
Anomaly detection in data for structural health monitoring
Structural health monitoring (SHM) has been extensively utilized in civil infrastructures for several decades. Various sensors have been used to monitor the status of civil constructions. Nevertheless, there are some abnormalities, like extreme weather, faulty sensors, and structural damage, that affect data accuracy. Notability: even cutting-edge damage diagnostic methods only apply with a proper date-cleansing process. It is vital to detect these abnormalities quickly to achieve successful civil structure monitoring.
A recent study published on “sensors” proposes using a hyperparameter-optimized Convolutional Neural Network (CNN) to address multiclass anomaly detection in an imbalanced dataset. The study assesses the effectiveness of a 1D CNN model using real-world multiclass time series data from a cable-stayed bridge with anomalies. Additional data is incorporated as required to ensure a balanced dataset. This study presented a fresh SHM method that yielded an approximate accuracy of 98% on the test data. For more information, you can access the article by link bellow.
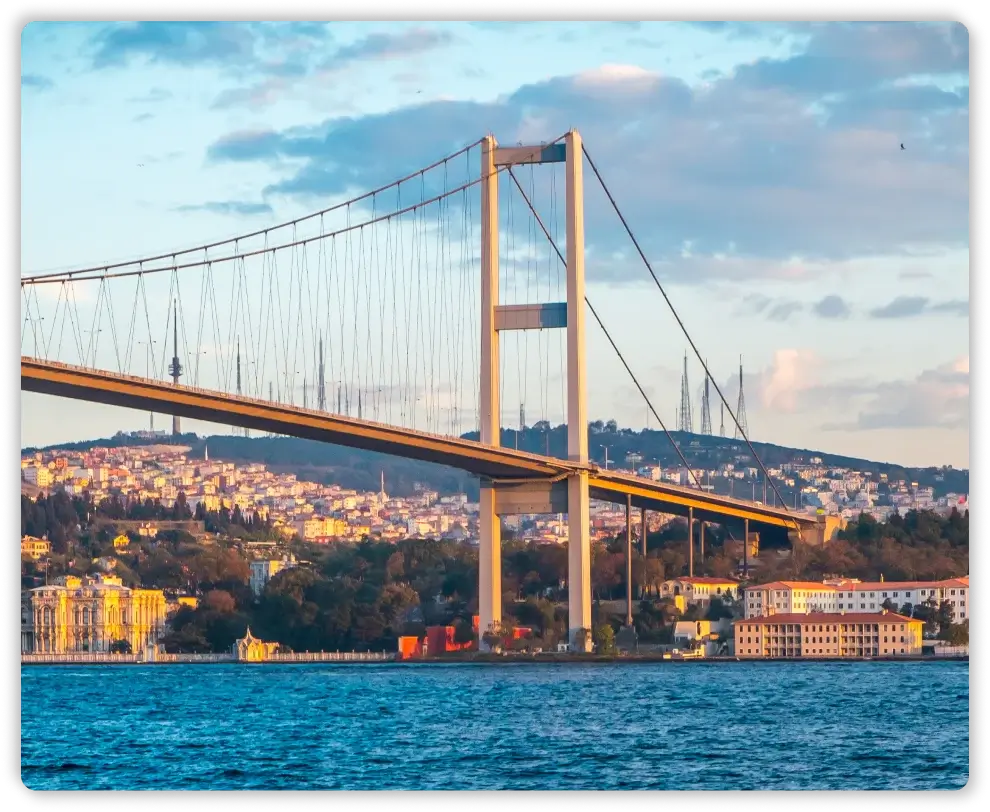